Associates At Biglaw Firm Now Really Bringing In The Dough
Poppin' fresh salary news.
He-hee.
Nothing says lovin’ like salary increases in the oven. It is this tortured series of references that bring us to the news that Pillsbury has matched the prevailing salary scale.
After flirting with a merger earlier this year, Pillsbury is closing out the year with some good news for its attorneys.
The firm follows a slightly different classification system than most:
Class | Bonus |
First-years | $225K |
Second-years | $235K |
Third-years | $260K |
Fourth-years | $310K |
Fifth-years | $365K |
Senior Associate 1 | $390K |
Senior Associate 2 | $420K |
Counsel | $435K |
As for bonuses, the firm commits to maintaining its established market bonus and super bonus formula. So that’s a match based on the corresponding class year and, if the past remains the guide, an additional $50,000 on top of these numbers for top performers.
Memo on the next page.
Please help us help you when it comes to salary news at other firms. As soon as your firm’s memo comes out, please email it to us (subject line: “[Firm Name] Salary”) or text us (646-820-8477). Please include the memo if available. You can take a photo of the memo and send it via text or email if you don’t want to forward the original PDF or Word file.
And if you’d like to sign up for ATL’s Salary & Bonus Alerts, please scroll down and enter your email address in the box below this post. If you previously signed up for the bonus alerts, you don’t need to do anything. You’ll receive an email notification within minutes of each bonus announcement that we publish.
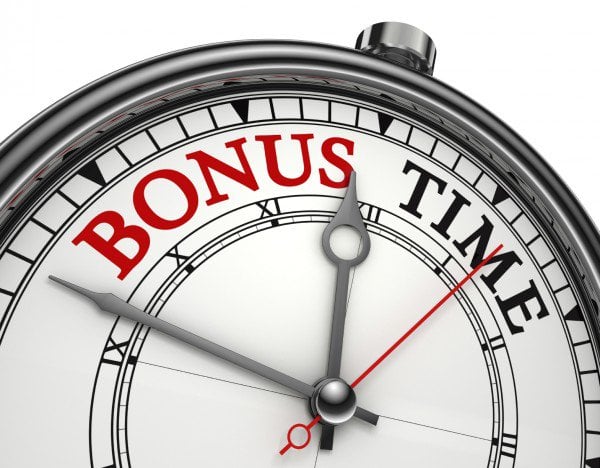